Finding Berries: Segmentation and Counting of Cranberries using Point Supervision and Shape Priors
Proceedings of the IEEE Conference on Computer Vision and Pattern Recognition Workshops, 2020 (Oral Presentation)
Peri Akiva, Kristin Dana, Peter Oudemans, Michael Mars
Overview of pipeline. Left: scene illustration of data collection stage. Top right: image captured by the drone. Bottom right: segmentation and count outputs of our Triple-S network. Colors in prediction mask are random and are used to represent instances (colors may repeat). Best viewed in color and zoomed.
Abstract
Precision agriculture has become a key factor for increasing crop yields by providing essential information to decision makers. In this work, we present a deep learning method for simultaneous segmentation and counting of cranberries to aid in yield estimation and sun exposure predictions. Notably, supervision is done using low cost center point annotations. The approach, named Triple-S Network, incorporates a three-part loss with shape priors to promote better fitting to objects of known shape typical in agricultural scenes. Our results improve overall segmentation performance by more than 6.74% and counting results by 22.91% when compared to state-of-the-art. To train and evaluate the network, we have collected the CRanberry Aerial Imagery Dataset (CRAID), the largest dataset of aerial drone imagery from cranberry fields. This dataset will be made publicly available.
CRanberry Aerial Imagery Dataset (CRAID)
Full dataset contains 21,436 cranberry images of size 456x608. Images were collected using a Phanthon 4 drone from a smal range of altitutdes with manually fixed camera settings: 100 ISO, 1/240 shutter speed, and 5.0 F-Stop. Data was collected at weekly intervals to capture albedo variations in cranberries.
Annotation procedure include center points and berry-wise annotations. Center points locate cranberry centers with equal number of background points. Background points are annotated at random locations, as far as possible from nearby cranberry annotations. Berry wise annotations are fully supervsied labels tht account for cranberry occlusion. While only visible cranberries are labeled, if there is an occluded part, it is estimated.
CRAID has an average of 39.22 cranberries per image, with minimum count of 0 and maximum count of 167. A single image covers roughly 1 square foot area.
The dataset is available through this link.
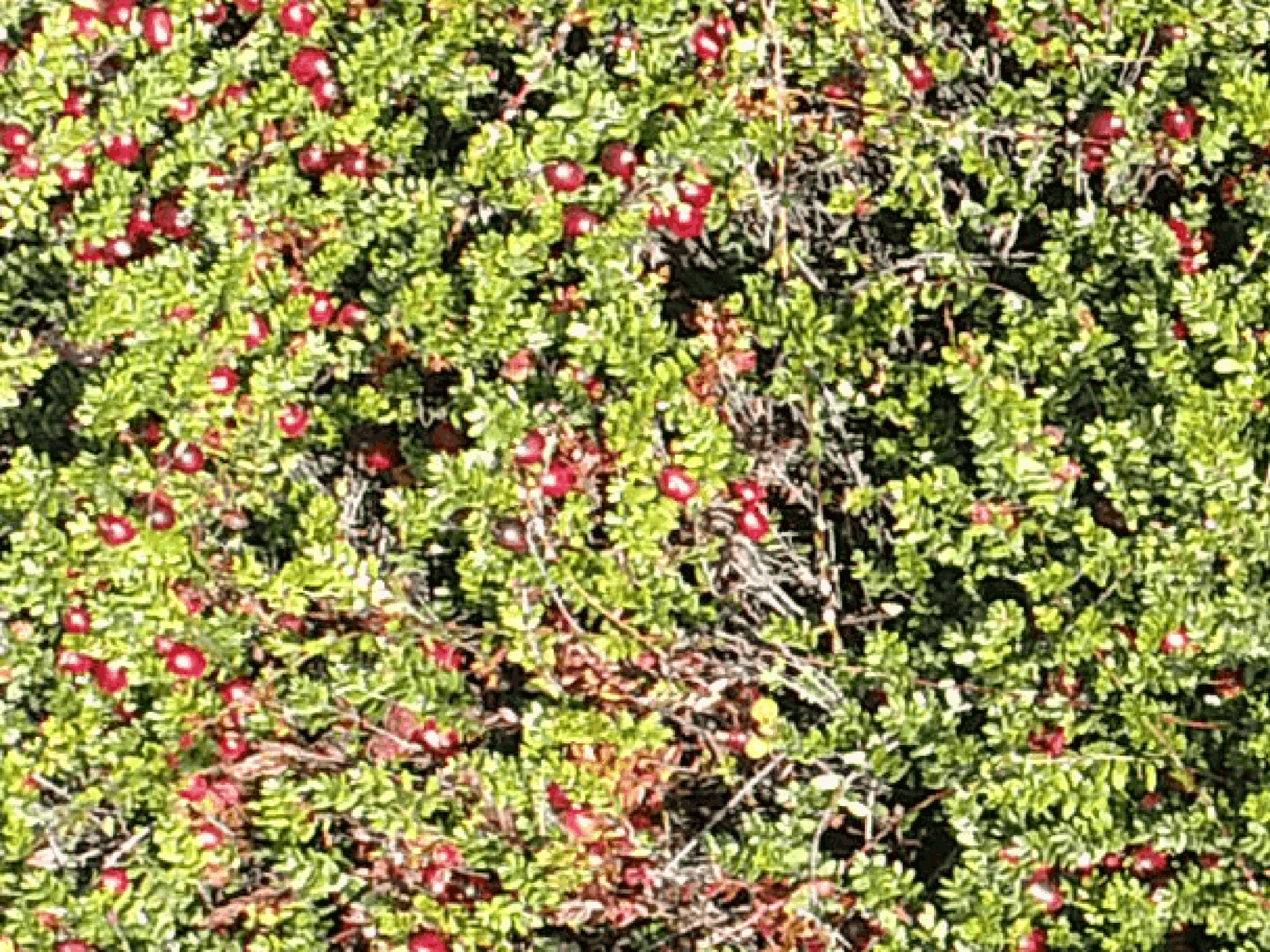
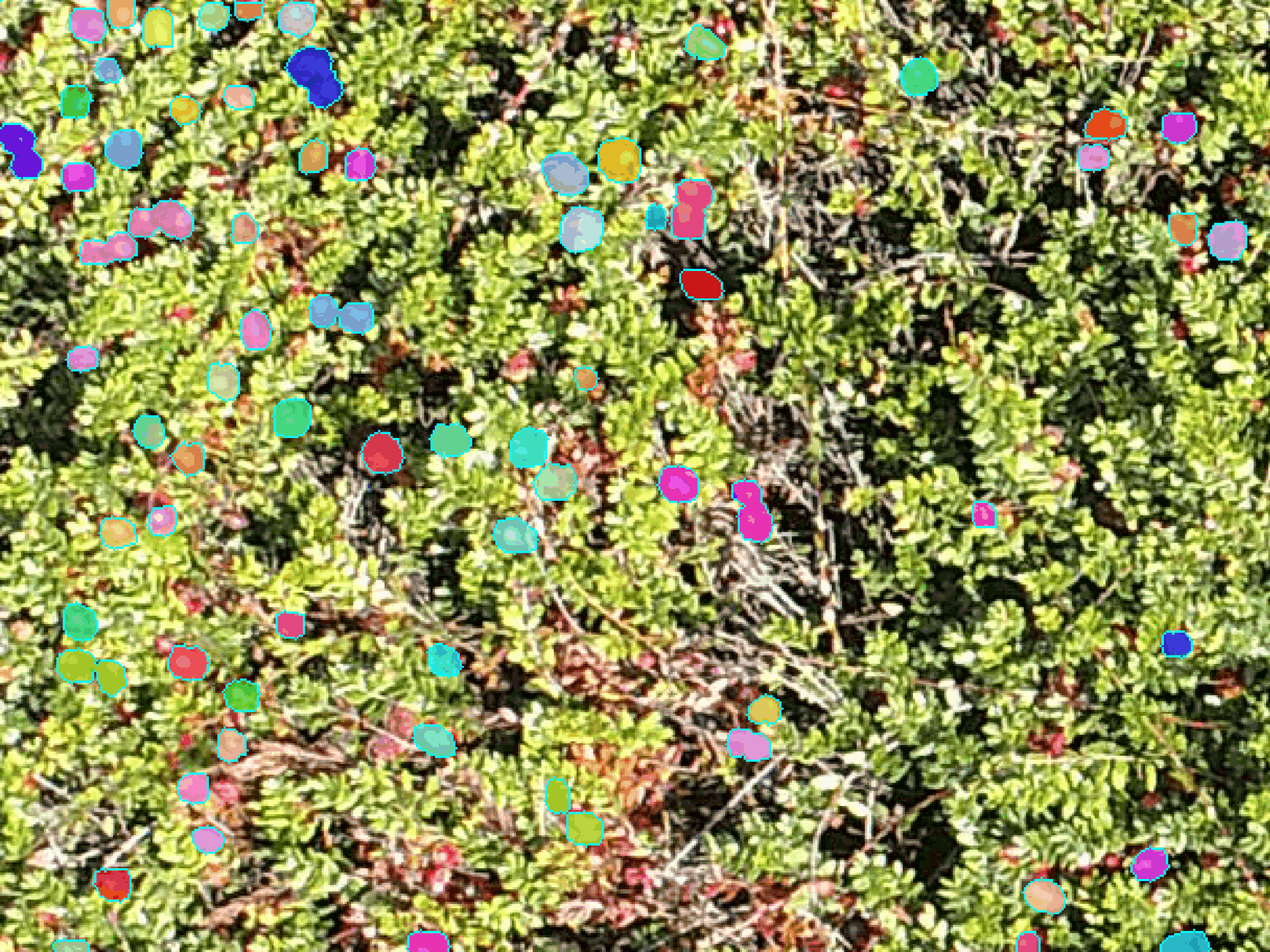
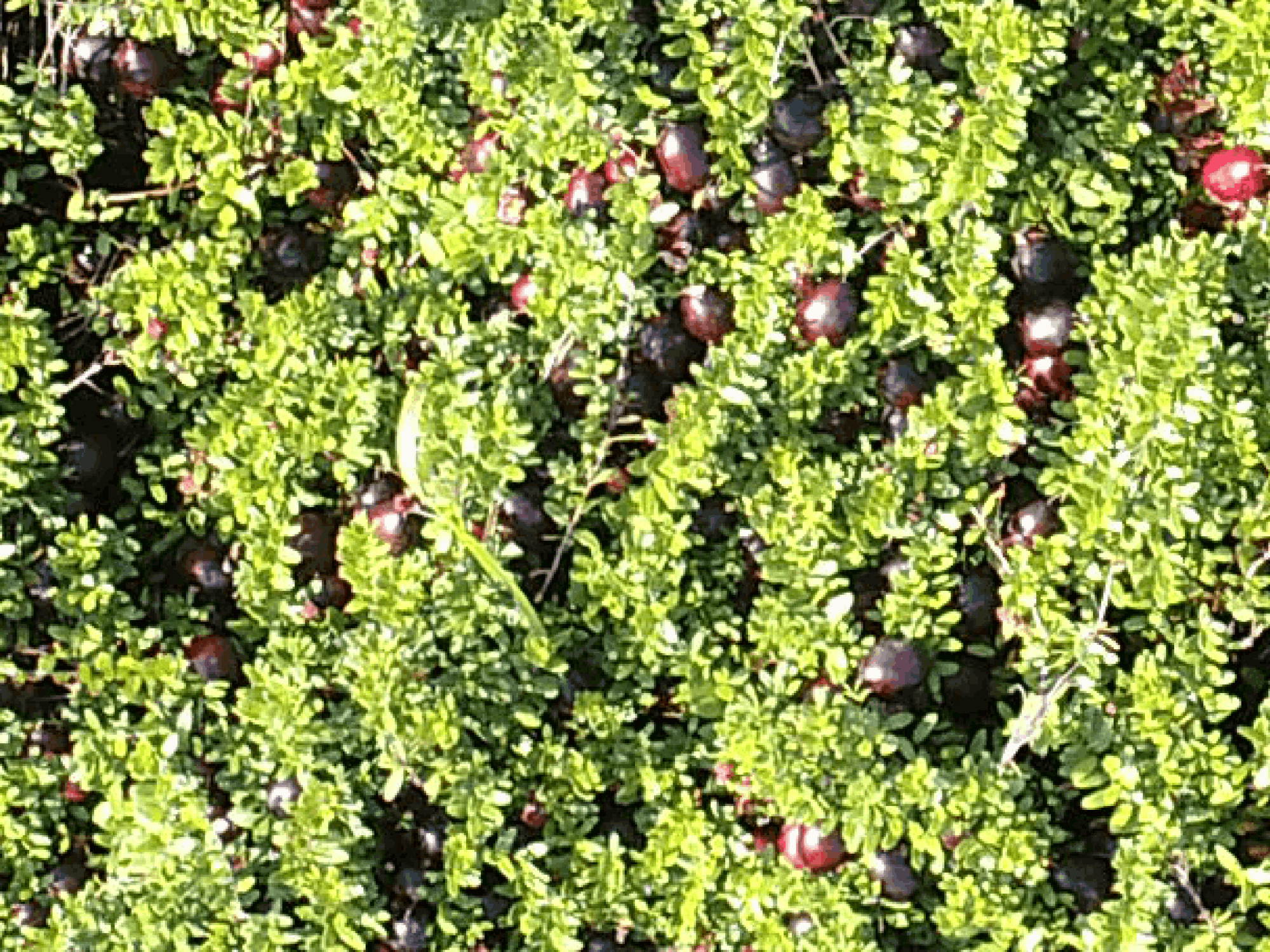
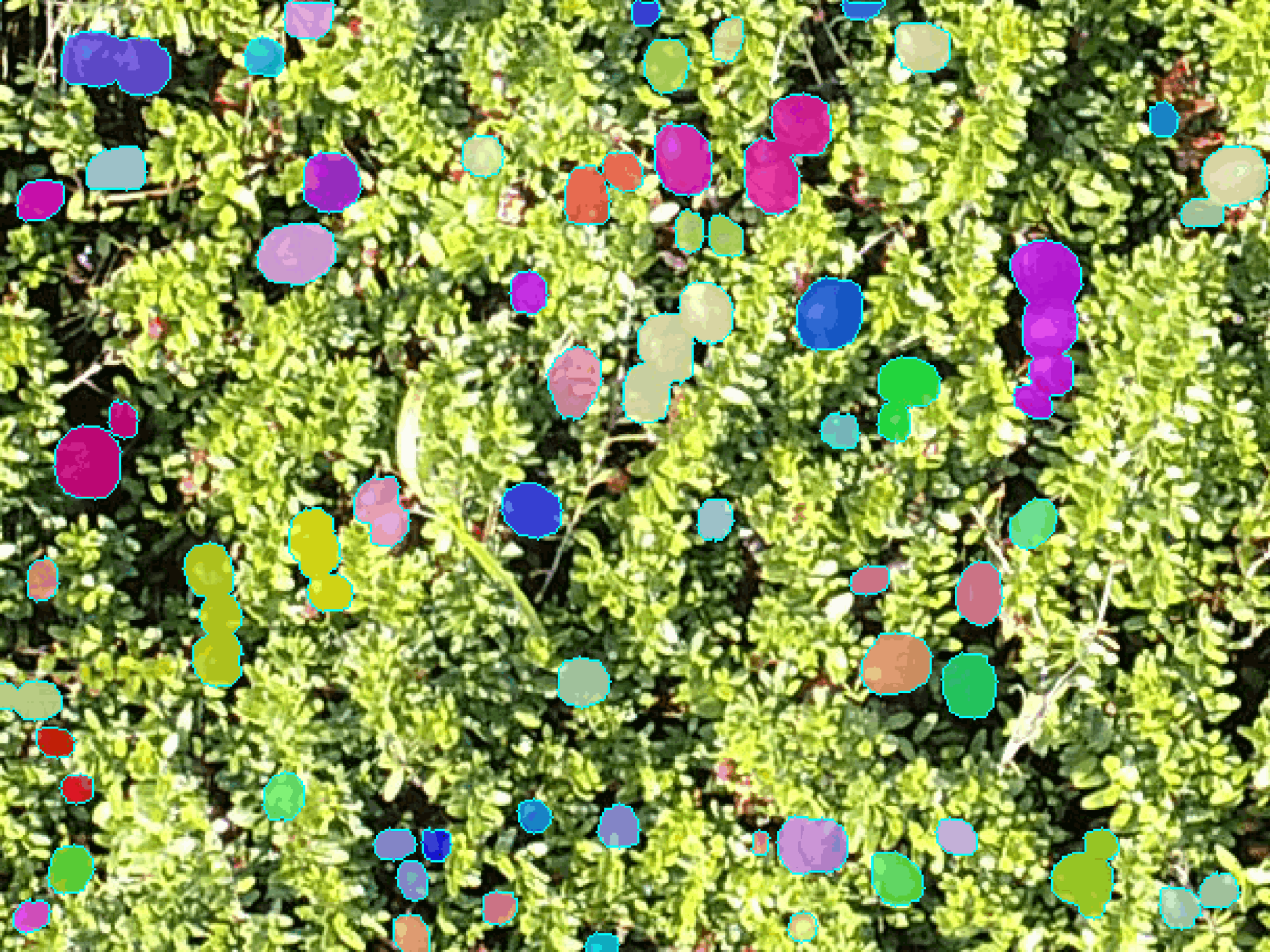
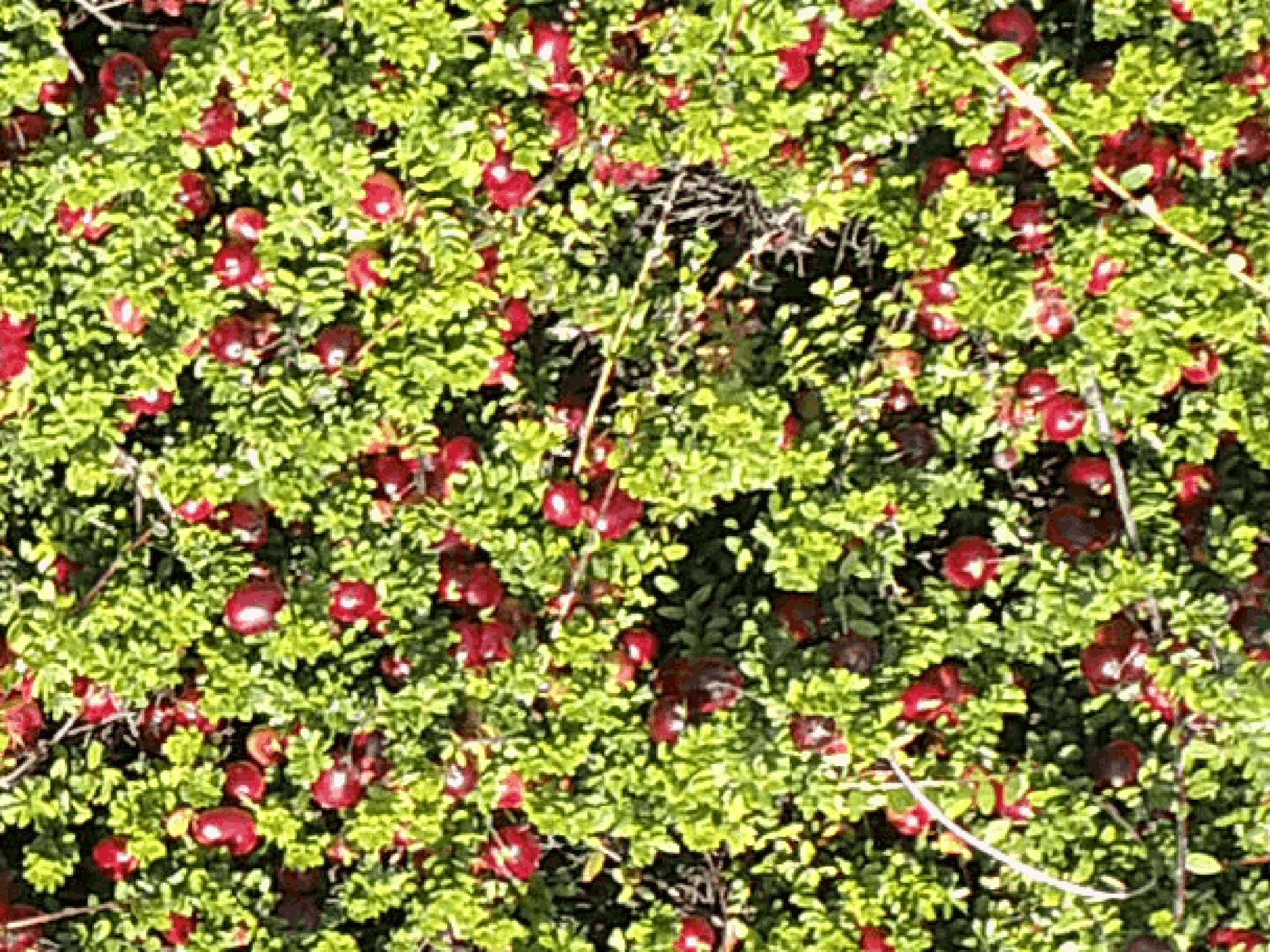
Image
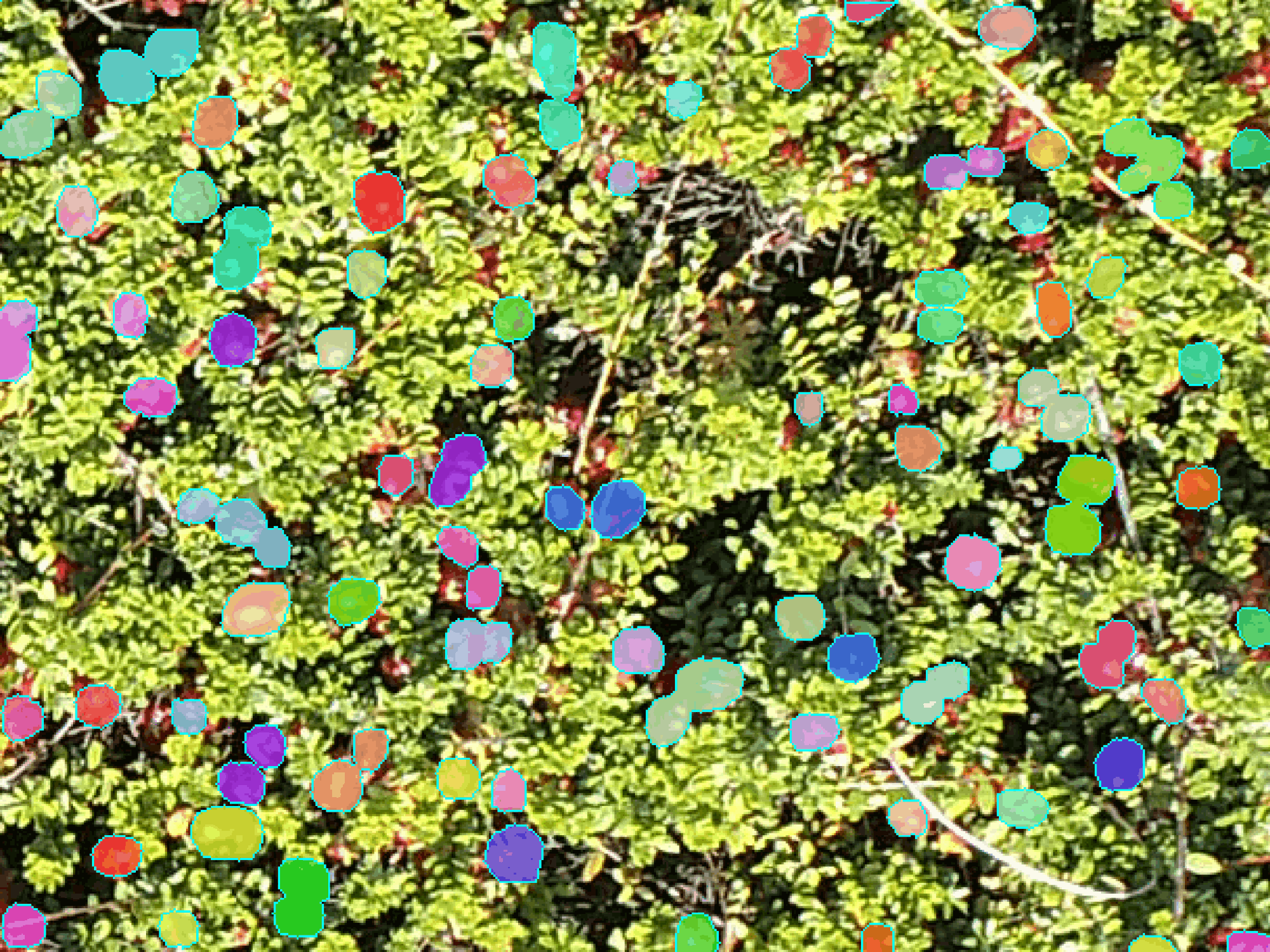
Hand labeled data
Video
Paper
@InProceedings{Akiva_2020_CVPR_Workshops,
title = {Finding Berries: Segmentation and Counting of Cranberries Using Point Supervision and Shape Priors},
author = {Akiva, Peri and Dana, Kristin and Oudemans, Peter and Mars, Michael},
booktitle = {The IEEE/CVF Conference on Computer Vision and Pattern Recognition (CVPR) Workshops},
year = {2020}